Robust clinically acceptable plans were produced by the method in under 20 minutes without any intervention from the user. This means that multiple plans easily can be generated using the powerful scripting functionality in RayStation”
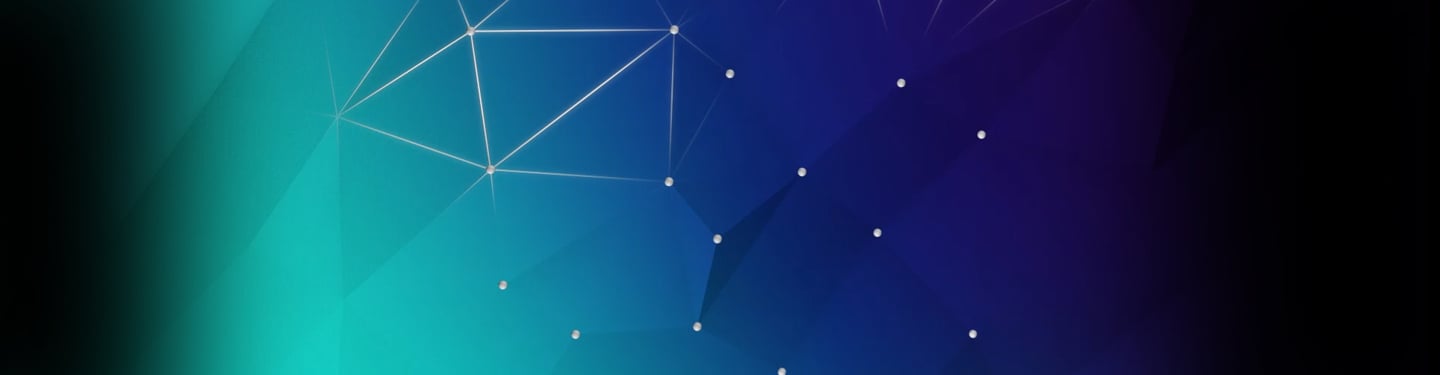
Case study: University Medical Center Groningen
AUTOMATED ROBUST PLANNING FOR IMPT IN HN CANCER USING MACHINE LEARNING
Proton therapy offers several advantages over other forms of radiation therapy. The main one is that this modality can deposit most of the energy from the radiation beams within the target volume. However, compared to conventional radiotherapy treatments, this type of radiation also presents challenges such as increased sensitivity to setup and density uncertainties.
Robust planning, released in RayStation 4.5 in 2014, has been developed to meet these challenges by providing high-quality treatment plans to handle any uncertainties. Nonetheless, manual planning of this type can be cumbersome. The aim of this study is to use machine learning to automate robust planning for intensity modulated proton (IMPT) plans.
ROBUST MACHINE LEARNING AUTOMATION
The clinicians at UMCG Proton Therapy Center are experts on proton planning and have adopted new technologies to ensure high levels of treatment quality and efficiency. With the objective of automating the creation of high-quality robust IMPT plans, UMCG and RaySearch partnered to evaluate and develop the very first machine learning planning algorithm that considers plan robustness on the same detailed level as a conventionally robust optimized plan.
The study focused on robust IMPT plans for oropharyngeal patients and used the machine learning automated treatment planning method that was released in December 2018. This algorithm learns from historical patient and plan data and infers a 3D spatial dose on a new patient geometry. The historical data used to create the machine learning model consisted of 80 oropharyngeal head and neck cancer patients, that were treated with IMPT the last two years at the UMCG Proton Therapy Center.
The objective of the planning for all patients was curative and the dose was optimized robustly in RayStation 8B to cover the clinical target volume (CTV) with an isotropic position uncertainty from 3 to 5 mm and a density uncertainty of 3%. The primary tumor CTV high had a dose prescription of 7,000 cGy and the bilateral elective lymph node regions CTV low were prescribed 5,425 cGy.
*Subject to regulatory clearance in some markets.
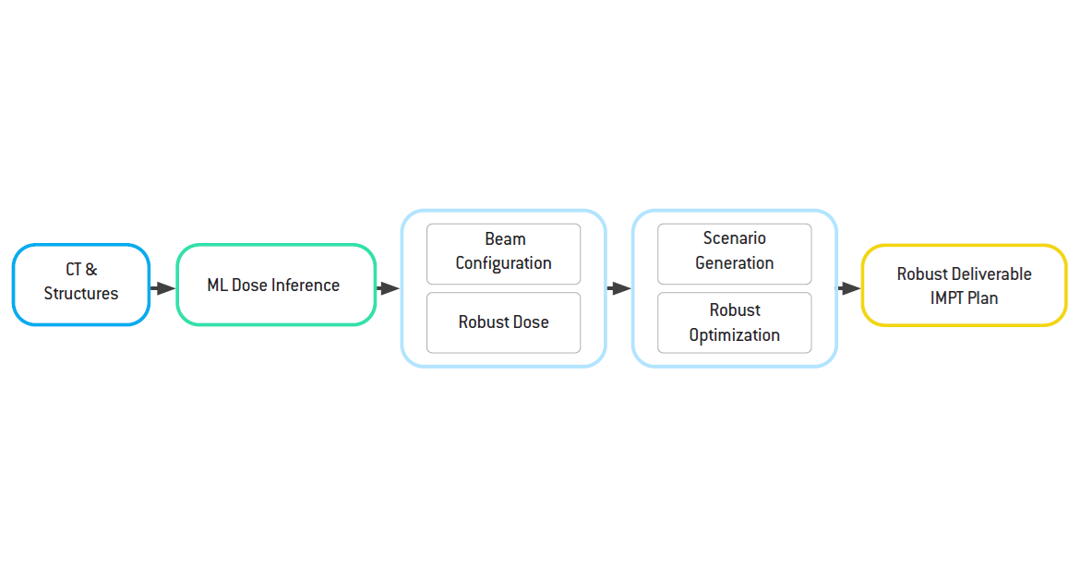
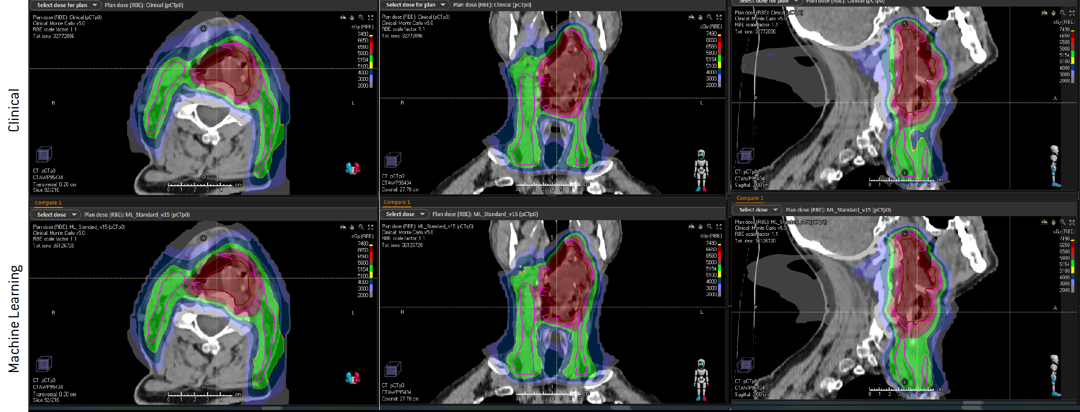
PLAN-GENERATION FRAMEWORK
The machine learning planning framework in this study consists of a machine learning algorithm that infers a robust dose distribution for a novel patient, given CT as well as structures and then performs robust optimization with the goal of mimicking the machine learning dose. Multiple scenarios, the beam configuration, and other physical constraints are considered by the robust optimization. Effectively, this framework produces an IMPT plan for the configured delivery. This optimization can be applied for several treatment techniques, such as pencil beam scanning.
Robust clinically acceptable plans were produced by the method in under 20 minutes without any intervention from the user. This means that multiple plans easily can be generated using the powerful scripting functionality in RayStation, Erik Korevaar, Medical Physicist, says.
The clinical target coverage was evaluated by studying the voxel wise minimum dose across scenarios of the CTV for each plan.
Furthermore, by studying the NTCP values on the nominal doses for both dysphagia and xerostomia the OAR doses were deemed comparable to those of the historical plans.
Table 1 shows the resulting toxicity and dose values in comparison between a clinical IMPT plan and the machine learning IMPT plan for a representative patient. Figure 1 shows the dose for the patient, both the robustly mimicked machine learning plan, and the clinical plan. Moreover, Figure 2 depicts the plan-robustness analysis of the clinical and machine learning plans in which DVH curves are shown for a large number of setup- and density-error scenarios. In the evaluation, the isotropic position, and a density uncertainty, were analogous to that of the clinical plans used for training of the machine learning algorithm. Both spatial dose visualization and DVH comparison for scenarios demonstrate that the machine learning generated IMPT plan is of clinical quality.
Plan | NTCP Grade 2 Xerostomia | NTCP Grade 2 Dysphagia | NTCP Grade 3 Xerostomia | NTCP Grade 3 Dysphagia | CTV high D98 voxelmin |
CTV low D98 voxelmin |
Clinical | 39.47 | 18.04 | 10.64 | 10.54 | 6662 | 5135 |
ML | 37.33 | 16.83 | 9.93 | 9.26 | 6635 | 5118 |
Table 1. NTCP and target dose for a clinical IMPT plan and a machine learning IMPT plan.
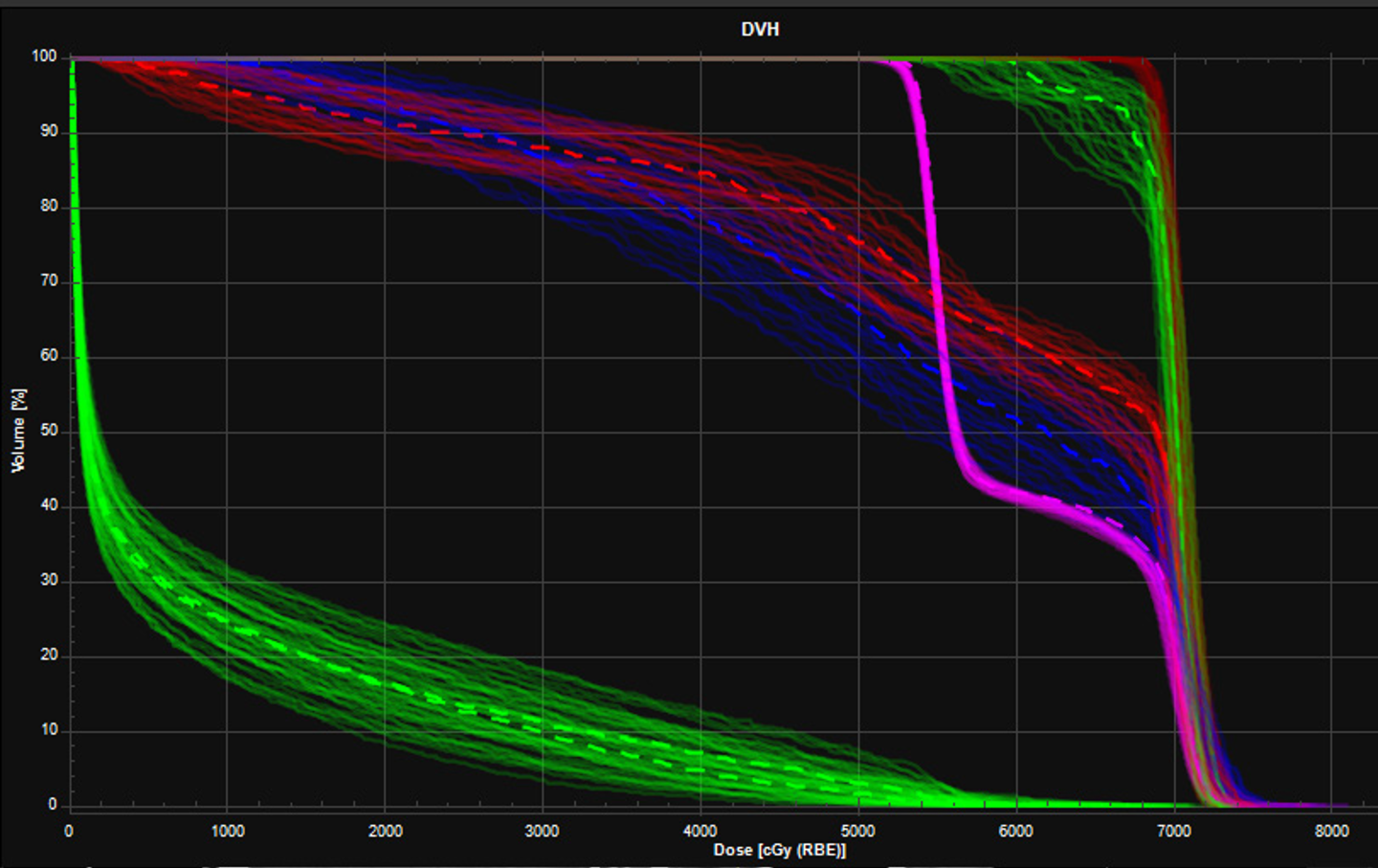
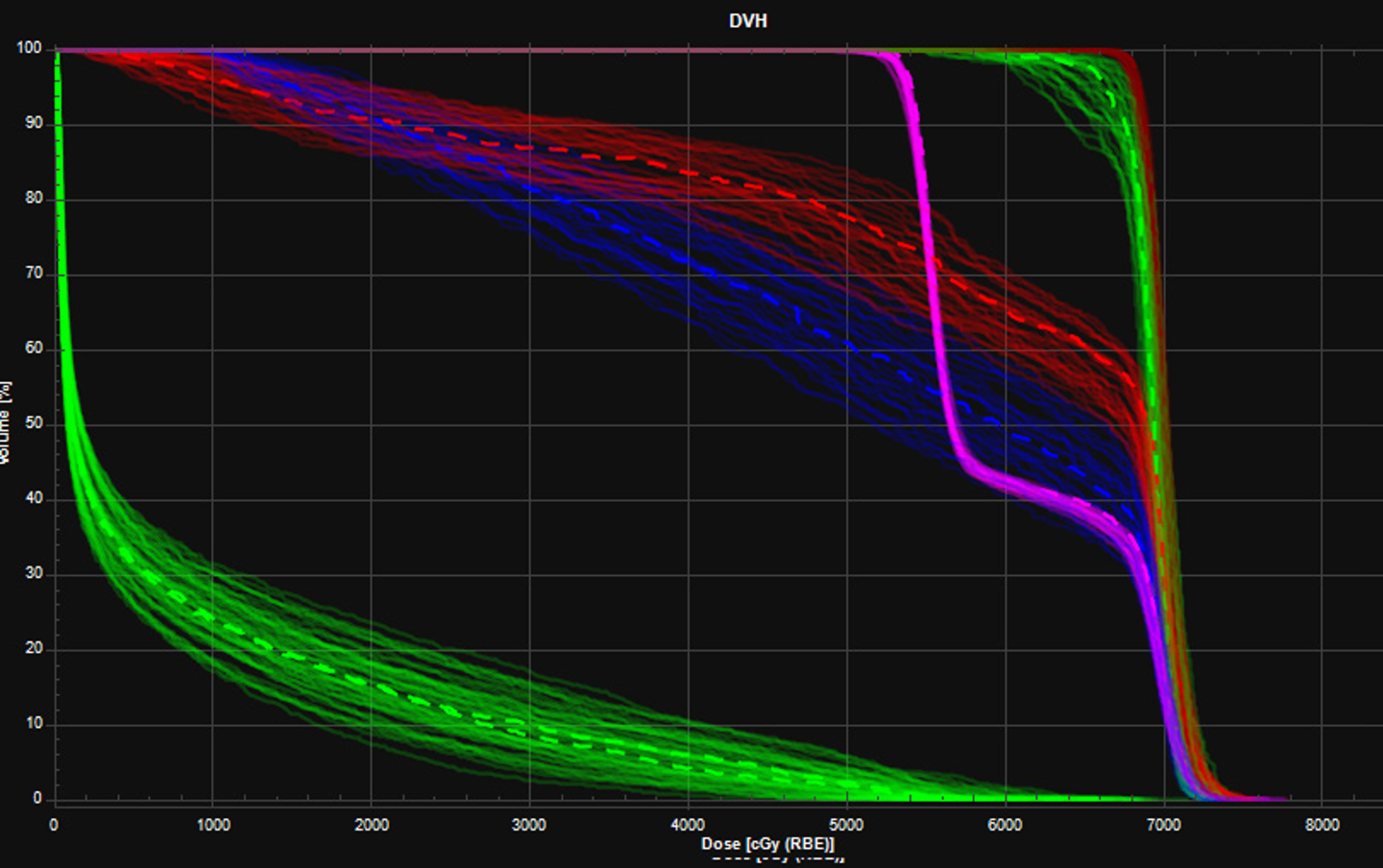
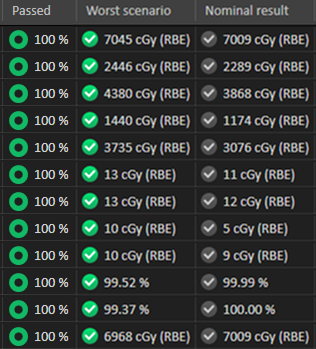
Moreover, Figure 3 shows the clinical goal acceptance in the robustness evaluation for each plan. All the scenarios meet the clinical goals with the sole exception of the max average dose in CTV high.
EFFICIENCY GAINS AND POTENTIAL FOR THE FUTURE
The machine learning generated plan for a representative patient was comparable to the clinical plan in terms of clinical goals and robustness. This possesses great potential in clinical practice as machine learning can produce robust plans with significant reduction to manual workloads. It can therefore be leveraged to produce quicker radiation therapy treatment planning or used in plan comparison for protons and photons. In the Netherlands, NTCP-based modality selection was applied for new patients and this machine learning framework enabled the automatic creation of both photon and proton plans, which can shorten the time patients have to wait for their first treatment. The overall framework applied in this study can also facilitate non-robust plan generation, which has been available for photons clinically since 2018. Additionally, the automation of IMPT treatment plan creation promises significant benefits for applications of adaptive radiation therapy, where quick re-planning is essential.
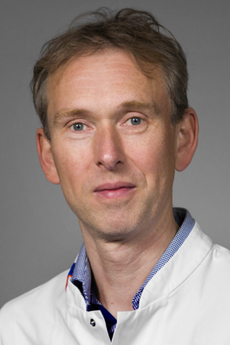
ADVANCING CANCER TREATMENT
RaySearch is a committed pioneer of oncology software. Since 2000, we have worked in close cooperation with leading centers to improve life and outcomes for patients. We develop all our products from the ground up and continuously revise every aspect, from algorithms to user interface designs. Medical science never stands still, and neither does RaySearch — our relentless drive to do things better leads us to ever-higher performance, accuracy, safety and usability. And this is just the beginning.
We believe software is the driving force for innovation in oncology. Our systems use groundbreaking automation and machine learning to create new possibilities. RayCare*, the next-generation oncology information system, will enable one workflow for all the oncology disciplines, ensuring fluid coordination of tasks and optimal use of resources. RayStation harmonizes treatment planning, providing one point of control for all planning needs — any equipment, any scale.
*Subject to regulatory clearance in some markets.
For more information or to see a demo, contact sales@raysearchlabs.com